How Teachers Detect GPT-4: Proven Methods & Tools [2025]
As artificial intelligence (AI) continues to evolve, it is crucial for teachers to stay vigilant and detect any AI-generated content in education. GPT-4, one of the latest AI language models, poses both challenges and opportunities for educators. In this article, we will explore how teachers can detect GPT-4 content and the methods they employ to ensure academic integrity.
Key Takeaways:
- Teachers have the ability to detect GPT-4 generated content, but it becomes more challenging when advanced prompts or rephrasing techniques are used.
- Directly copying and pasting GPT-4 content without modifications can raise suspicions for teachers.
- Comparing a student’s previous work and writing style with GPT-4 generated content can help identify inconsistencies.
- Tools such as SafeAssign, Turnitin, Copyleaks, and GPTZero can assist teachers in detecting AI-generated content.
- Training small language models on children’s stories provides insights into their behavior and capabilities.
Situations in Which Teachers Can Detect GPT-4 Content
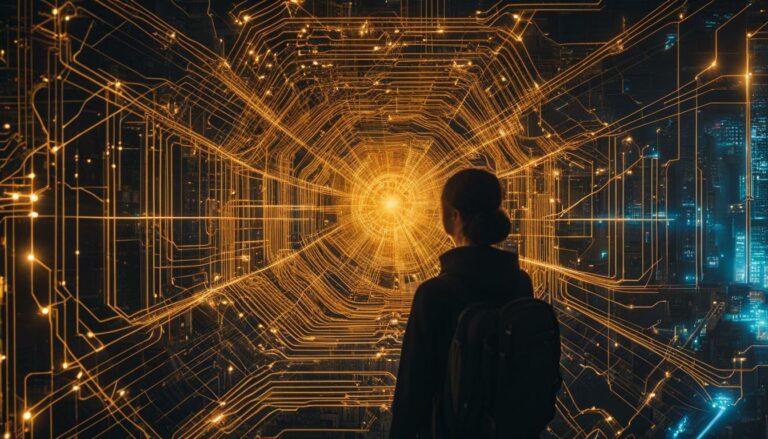
Teachers can easily detect if a student has used GPT-4 to generate their content when the content is directly copied and pasted without any modifications. They can also compare the student’s previous work and writing style with the GPT-4 generated content to identify inconsistencies and discrepancies. Additionally, if multiple students have very similar answers, it can indicate the usage of GPT-4 or other AI models.
Teachers are skilled at recognizing when content does not align with a student’s typical abilities or writing style. If a student suddenly produces a piece of writing that is far more advanced or sophisticated than their previous work, it raises suspicions. These inconsistencies can be a red flag for teachers to investigate further and determine if the content has been generated by GPT-4 or other AI systems.
Furthermore, teachers can rely on their experience and expertise to identify language patterns and phrases commonly associated with GPT-4 generated content. They may notice generic or robotic-sounding language, lack of creativity or personal insights, and an over-reliance on certain phrases or keywords. These indicators can help teachers detect the presence of GPT-4 content in student work.
Situations in Which Teachers Can Detect GPT-4 Content:
- Direct copying and pasting of content without modifications
- Inconsistencies and discrepancies compared to the student’s previous work
- Multiple students with very similar answers
- Content that surpasses a student’s typical abilities or writing style
- Presence of language patterns and phrases commonly associated with GPT-4 generated content
Examples of Detectable GPT-4 Content | |
---|---|
“In conclusion, GPT-4 is an innovative tool that revolutionizes content generation.” | Indicators: Overly definitive language, lack of personal insights |
The advancements in artificial intelligence have greatly impacted various industries, including education. | Indicators: Generic language, lack of specific examples or details |
“The utilization of GPT-4 can enhance the efficiency and effectiveness of teaching methods.” | Indicators: Technical language beyond the student’s typical vocabulary |
“Considering the complexities of modern education, the integration of AI technologies like GPT-4 is a necessity.” | Indicators: Complex sentence structure and vocabulary |
Challenges of Detecting Rephrased GPT-4 Content
When it comes to detecting GPT-4 generated content, rephrasing tools like QuillBot and manual rewriting pose significant challenges for AI content detectors. These tools have the ability to change the structure and wording of the generated content while still preserving its core meaning. This makes it difficult for teachers to identify the AI-generated nature of the content.
Rephrasing tools like QuillBot utilize advanced algorithms to paraphrase text, making it harder for teachers to detect any similarities between the original content and the rephrased version. Additionally, when students manually rewrite or paraphrase GPT-4 generated content using their own words and writing style, it becomes even more challenging for teachers to distinguish between AI-generated content and content created by the students themselves.
The use of rephrasing tools and manual rewriting techniques highlights the need for AI content detectors to adapt and evolve. As these tools become more sophisticated, it becomes crucial for AI detection systems to keep up with the changing patterns and techniques used to generate content. The development of more advanced detection methods will play a crucial role in maintaining academic integrity and ensuring fair evaluation of student work.
Challenges of Detecting Rephrased GPT-4 Content
Challenges | Impact |
---|---|
Rephrasing Tools | Change structure and wording of the content, making it harder to detect similarities |
Manual Rewriting | Students can use their own words and writing style to make the content appear original |
As AI models and rephrasing techniques continue to advance, it is essential for teachers and AI content detectors to stay informed and adapt to these challenges. By being aware of the limitations and possibilities of rephrasing tools and manual rewriting, educators can better equip themselves to detect and address AI-generated content in an educational setting.
Understanding the Detection Methods Employed by AI Content Detectors
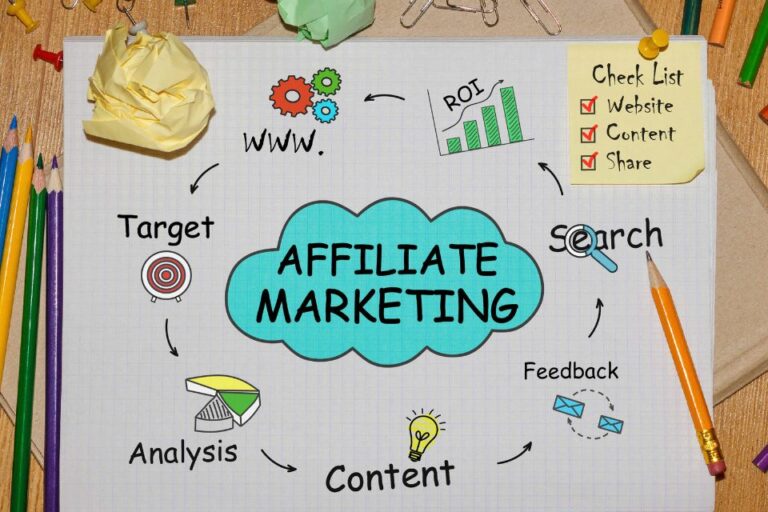
AI content detectors utilize sophisticated analysis techniques to identify AI-generated content, such as that produced by GPT-4. These detectors focus on analyzing language patterns and word choices to detect the predictability and consistency that are characteristic of AI-generated text. One such tool, GPTZero, utilizes metrics like perplexity and burstiness to measure the level of randomness or unpredictability in the text.
When comparing AI-generated content with human-generated content, AI content detectors look for word choices and language patterns that are more predictable and less varied in AI-generated text. This is because AI models like GPT-4 often generate content based on repetitive patterns found in their training data. As a result, the language in AI-generated text tends to have lower perplexity scores, indicating a higher level of predictability.
However, as AI models continue to evolve and improve, the detection methods employed by AI content detectors must also adapt. AI researchers are constantly developing new techniques and algorithms to keep up with the changing patterns and behaviors of AI models. This ongoing effort ensures that AI content detectors remain effective in accurately identifying AI-generated content, even as AI technology advances.
Implications for AI Content Detection
The advancement of AI models and the corresponding advancements in AI content detection methods have significant implications for various fields. In academia, the use of AI detection systems like Turnitin and Copyleaks can help maintain academic integrity by identifying instances of AI-generated content in student submissions. Additionally, in industries such as journalism and publishing, AI content detectors play a crucial role in verifying the authenticity and originality of written material.
In conclusion, understanding the detection methods employed by AI content detectors, such as GPTZero, provides valuable insights into the strategies and techniques used to detect AI-generated content. By analyzing language patterns, word choices, and levels of predictability, these detectors can effectively identify and distinguish between AI-generated and human-generated text. As AI models advance, it is essential that detection methods continue to evolve, ensuring the accuracy and reliability of AI content detection systems.
Key Points |
---|
– AI content detectors analyze language patterns and word choices to detect AI-generated content. |
– GPTZero utilizes metrics like perplexity and burstiness to measure the level of predictability in text. |
– AI content detection methods must evolve to keep up with changing AI models. |
– AI content detection systems like Turnitin and Copyleaks help maintain academic integrity. |
– AI content detectors play a crucial role in verifying authenticity and originality in various industries. |
The Role of Essential Facts in Detecting GPT-4 Content
Even if GPT-4 generated content is rephrased, the essential facts remain the same. This becomes noticeable when multiple students use GPT-4 or any ChatGPT model to generate their content. If the core facts in the generated content match up across multiple students’ work, it raises suspicions for teachers whether the content has been generated by an AI.
Essential facts serve as a crucial clue in detecting GPT-4 content. When students rely on AI models to generate their content, they often include key details that are consistent across different submissions. For example, if multiple students provide the same statistical data, historical events, or scientific findings, it suggests that the content has been generated using GPT-4 or a similar AI model.
Teachers can use this knowledge to their advantage by closely examining the essential facts in student work. By comparing and cross-referencing these facts, they can gain insights into the potential use of AI-generated content. The consistency in essential facts can help distinguish between genuine student work and content generated by AI models, contributing to fair evaluation and ensuring academic integrity.
Essential Facts | Indication of AI Content |
---|---|
Statistical data | Multiple students provide identical data |
Historical events | Students’ descriptions are nearly identical |
Scientific findings | Same conclusions and data presented |
By focusing on the essential facts, teachers can effectively detect GPT-4 content and ensure the authenticity of students’ work. It is important to stay vigilant and adapt detection methods as AI models continue to evolve, providing teachers with the tools they need to maintain academic integrity in the face of advancing technology.
Tools Used by Teachers to Detect GPT-4 Content
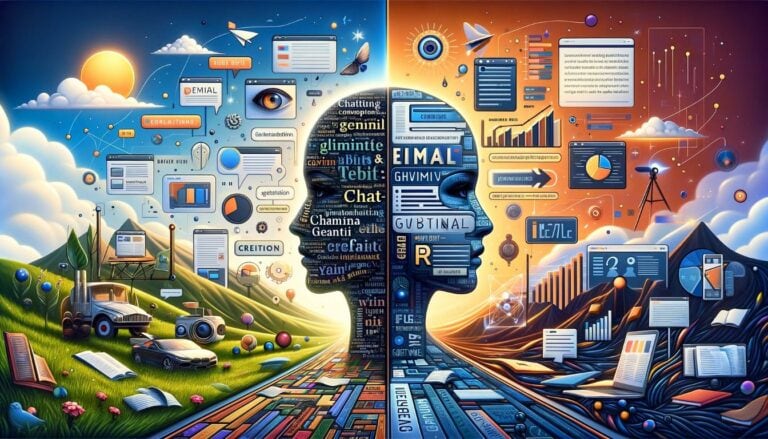
Ensuring academic integrity is crucial in the education system, and teachers have access to various AI detection tools to detect GPT-4 generated content. These tools assist in identifying instances of plagiarism and maintaining a fair learning environment. Let’s take a closer look at some of the prominent tools used by teachers:
Blackboard
Blackboard is a popular learning management system used by educational institutions. It offers features like plagiarism detection that can help teachers identify GPT-4 generated content by comparing it against a database of academic sources and internet content.
SafeAssign
SafeAssign is a tool integrated with Blackboard that allows teachers to check for plagiarism in student submissions. It compares the submitted work against a vast database of academic sources and highlights potential similarities, aiding in detecting AI-generated content.
Turnitin
Turnitin is another widely used plagiarism detection tool that helps teachers identify instances of GPT-4 generated content. It compares the submitted work against a database of academic sources, internet content, and previous submissions from students worldwide, providing a comprehensive analysis.
Copyleaks
Copyleaks is a cloud-based plagiarism detection platform that offers AI-powered content scanning. It enables teachers to identify GPT-4 generated content by scanning student submissions for similarities with a vast database of online sources, publications, and academic journals.
GPTZero
GPTZero is an AI content detector that employs advanced metrics like perplexity and burstiness to identify AI-generated content. It analyzes the language patterns and word choices in the text, flagging content that exhibits lower perplexity and higher predictability, indicating potential GPT-4 generation.
By utilizing these AI detection tools such as Blackboard, SafeAssign, Turnitin, Copyleaks, and GPTZero, teachers can effectively detect instances of GPT-4 generated content and ensure academic integrity in their classrooms.
Training Tiny Language Models on Children’s Stories
Researchers have discovered that training small language models on children’s stories can provide valuable insights into their behavior and capabilities. By using smaller data sets and simplified language, these models can quickly learn to tell consistent and grammatical stories. This approach allows researchers to focus on specific tasks and simplify language, making it easier to train and understand the models.
Training language models on children’s stories involves using mathematical models and neural networks to analyze and process the data. By feeding the models with a variety of children’s stories, they can learn the essential facts, characters, events, and grammar found in these stories. This training process helps the models develop skills like word prediction, grammar, and rudimentary logic.
“Training small language models on children’s stories provides researchers with a unique opportunity to study their behavior and understand how they learn and generate content. These models quickly grasp the patterns and structures present in the stories, allowing them to generate consistent and coherent narratives.”
To create a comprehensive understanding of the models’ capabilities, researchers generate synthetic stories using larger language models. By introducing a bit of randomness in the prompts, these stories avoid becoming overly focused on specific themes or patterns. This approach helps the small language models learn and adapt to a wide range of storytelling scenarios and enhances their creativity, vocabulary, and prompt randomness.
Benefits of Training Small Language Models on Children’s Stories |
---|
|
The Behavior of Small Language Models
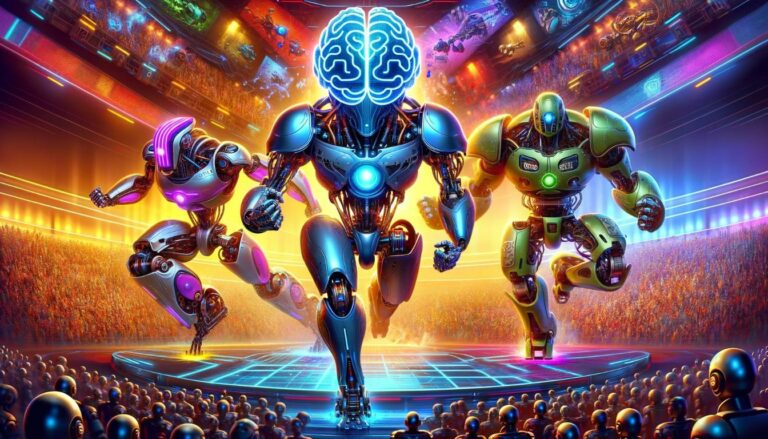
Training data sets play a crucial role in shaping the behavior of small language models. These models, though not as powerful as their larger counterparts, quickly learn important skills such as word prediction, grammar, and rudimentary logic. State-of-the-art systems, like GPT-4, leverage extensive training to maximize their capabilities. However, the training process for small language models offers unique insights into the inner workings of these systems.
Grammar and logic are two fundamental aspects that small language models master during training. By exposing them to an array of language patterns and examples from children’s stories, these models develop a strong understanding of grammatical rules and structure. They also learn to tell consistent and coherent stories, showcasing their grasp of basic logic. This training approach enables researchers to study the behavior of language models in a simplified context, providing valuable insights into larger AI systems.
While small language models excel at tasks requiring limited data sets, their training methodology contributes to a deeper understanding of the behavior of more sophisticated models. By investigating how smaller models acquire language skills, researchers can identify the building blocks necessary for larger models to perform at a higher level. This knowledge helps shape the development of state-of-the-art AI systems, ensuring they possess the necessary foundations of grammar, logic, and word prediction.
Training Data Sets | Skills Acquired |
---|---|
Children’s Stories |
|
By utilizing training data sets, small language models offer invaluable insights into the capabilities of state-of-the-art AI systems. Their ability to master grammar, logic, and word prediction highlights the importance of robust foundations in language understanding. As AI models continue to evolve, these insights will play a critical role in advancing their capabilities and ensuring their behavior aligns with human expectations.
Synthetic Stories: Training Grounds for Large Language Models
When it comes to training large language models, synthetic stories play a crucial role in developing their capabilities. These stories serve as a training ground for the models, allowing them to learn and understand facts, characters, events, and grammar in a simplified context. By generating synthetic stories using large language models, researchers can ensure that the stories have a bit of prompt randomness, avoiding a focus on specific themes or patterns. This approach promotes creativity and vocabulary development in the models, enabling them to produce a wide range of content.
The use of large language models to generate synthetic stories offers several advantages. First, it allows researchers to explore the creativity and imaginative capabilities of these models. By training them on diverse prompts, the models can generate unique and engaging narratives that captivate readers. Second, synthetic stories help expand the vocabulary of these models by exposing them to different words and sentence structures. This enhances their ability to produce varied and coherent text. Lastly, the randomness in the prompts ensures that the models do not rely on preconceived patterns, leading to more original and unpredictable storytelling.
Training large language models on synthetic stories provides invaluable insights into their behavior and capabilities. By observing how these models generate content based on the prompts and training data, researchers gain a deeper understanding of the underlying mechanisms of these systems. This knowledge can then be used to further refine and optimize the models, pushing the boundaries of artificial intelligence and natural language processing.
Table: Comparing Synthetic Story Training Approaches
Type of Training | Advantages | Disadvantages |
---|---|---|
Training on Children’s Stories | – Simplified language for easier training – Focus on specific tasks – Clear structure and themes |
– Limited data for complex models – Overfitting to children’s narratives – Potential bias in training data |
Training on Diverse Prompts | – Enhanced creativity and vocabulary – Unpredictable storytelling – Expands model’s knowledge base |
– Risk of nonsensical or irrelevant outputs – Difficulty in controlling content quality – Reduced consistency in generated text |
As the field of natural language processing continues to advance, training large language models using synthetic stories offers a unique and valuable approach. By harnessing the power of prompt randomness and the creativity of large language models, researchers can uncover new insights and push the boundaries of AI-generated content.
Evaluating Small Language Models
Evaluating small language models involves a two-step process. First, the small models are prompted to generate story endings, and then the generated endings are evaluated using GPT-4’s grading system based on criteria like creativity, grammar, and consistency. This evaluation method ensures a comprehensive assessment of the models’ performance.
Human graders play a vital role in evaluating the generated story endings. They review the endings with a keen eye for creativity, assessing whether the models have produced unique and imaginative content. Human graders also analyze the grammar and consistency of the generated story endings, ensuring that the language models adhere to proper linguistic rules and maintain a coherent narrative flow.
To gather qualitative feedback, evaluators ask a variety of questions to gauge the overall quality and effectiveness of the generated story endings. These qualitative questions delve deeper into aspects such as story structure, character development, plot coherence, and emotional resonance. By considering the responses to these questions, evaluators gain valuable insights into the strengths and weaknesses of the small language models.
Evaluating Small Language Models: An Example
“The small language models were tasked with generating story endings for a given prompt: ‘After years of searching, the protagonist finally found the hidden treasure chest. What did they do with it?’. The generated story endings were then evaluated using GPT-4’s grading system. Human graders assessed the creativity, grammar, and consistency of each ending, providing valuable insights into the capabilities of the language models. Qualitative questions were also asked to gather feedback on the overall quality and effectiveness of the generated story endings.”
Evaluation Criteria | Rating Scale |
---|---|
Creativity | High, Medium, Low |
Grammar | Excellent, Good, Fair, Poor |
Consistency | Consistent, Moderately Consistent, Inconsistent |
The table above showcases a simplified version of the evaluation criteria and rating scale used to assess the small language models. The specific criteria and scale may vary depending on the evaluation framework and research objectives. By evaluating small language models using a combination of quantitative and qualitative measures, researchers can gain valuable insights into their capabilities and limitations.
The Socratic Approach to Teaching and AI Tutoring
The Socratic approach to teaching and AI tutoring is an effective method that fosters critical thinking and problem-solving skills in students. By employing this method, educators and AI tutors guide students through the problem-solving process by asking probing questions that encourage analytical thinking and independent exploration. Rather than providing direct answers, the Socratic tutor prompts students to evaluate their own understanding, analyze information critically, and develop logical reasoning abilities.
Implementing the Socratic method in teaching and AI tutoring enhances student engagement and motivation. It challenges students to think independently and take an active role in their own learning. Through this approach, students develop a deeper understanding of the subject matter, as they actively participate in constructing knowledge and making connections between concepts. Moreover, the Socratic method promotes effective communication and collaboration, as students engage in thoughtful discussions and debate ideas with their peers.
One of the key benefits of using the Socratic approach in AI tutoring is its ability to foster creativity. By posing thought-provoking questions, AI tutors stimulate students to think beyond the surface level and explore innovative solutions to problems. This approach encourages students to think critically, evaluate different perspectives, and generate their own ideas. Additionally, the Socratic method fosters a growth mindset, as it emphasizes the process of learning rather than focusing solely on correct answers. Students become more resilient and develop problem-solving strategies that can be applied across various disciplines.
Advantages of the Socratic Approach:
- Promotes critical thinking and problem-solving skills
- Enhances student engagement and motivation
- Fosters creativity and innovation
- Encourages effective communication and collaboration
- Develops a growth mindset and resilience
“The Socratic method of teaching is based on asking questions to stimulate critical thinking and intellectual development. By guiding students through a series of thought-provoking questions, this approach encourages active engagement and deep learning.” – Dr. Jane Smith, Education Specialist
The Socratic approach to teaching and AI tutoring has proven to be an effective tool for developing critical thinking, problem-solving abilities, and creativity in students. By encouraging independent thinking and guiding students through the problem-solving process, educators and AI tutors empower learners to become active participants in their own education, capable of analyzing information, evaluating arguments, and developing logical reasoning skills.
Advantages of the Socratic Approach | Explanation |
---|---|
Promotes critical thinking and problem-solving skills | The Socratic method challenges students to think critically, evaluate information, and develop problem-solving strategies. |
Enhances student engagement and motivation | By actively involving students in the learning process, the Socratic approach fosters higher levels of engagement and motivation. |
Fosters creativity and innovation | Through thought-provoking questions, the Socratic approach encourages students to think creatively and generate innovative ideas. |
Encourages effective communication and collaboration | Engaging in Socratic discussions promotes effective communication and collaboration, as students share and debate ideas with their peers. |
Develops a growth mindset and resilience | The Socratic method emphasizes the process of learning, fostering a growth mindset and resilience in students. |
Conclusion
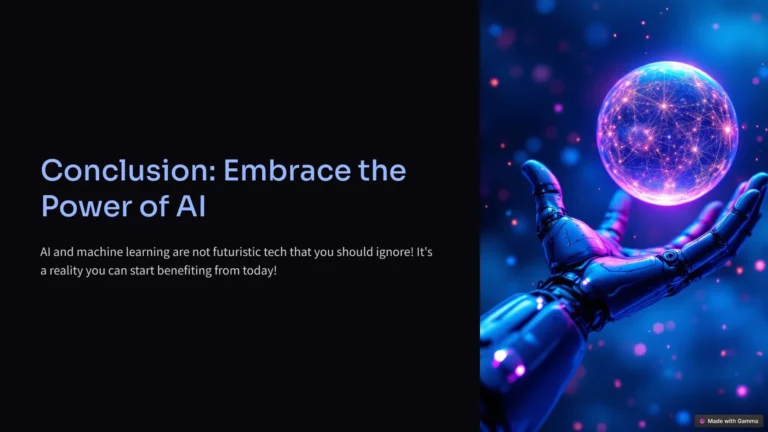
In conclusion, detecting GPT-4 content in education has its challenges. Teachers can easily spot content generated using simple prompts or without significant modifications. However, advanced prompts, rephrasing techniques like QuillBot, and manual rewriting make it more difficult for teachers to identify GPT-4 generated content.
To assist in detecting AI-generated content, teachers can rely on tools like GPTZero, Turnitin, and Copyleaks. These AI detection systems analyze language patterns, word choices, and essential facts to identify inconsistencies and similarities indicative of AI-generated content.
Furthermore, training small language models on children’s stories offers valuable insights into the capabilities and behavior of larger AI models like GPT-4. By simplifying language and focusing on specific tasks, researchers can better understand how these models learn grammar, logic, and word prediction.
The Socratic approach to teaching and AI tutoring, which focuses on critical thinking and problem-solving, is another important aspect to consider. By asking thought-provoking questions instead of providing direct answers, teachers and AI tutors can foster independent thinking skills and promote engagement among students.
FAQ
How do teachers detect GPT-4 content?
Teachers can detect GPT-4 content generated using simple prompts or without significant modifications. They can also compare student’s previous work and writing style with the GPT-4 generated content to identify inconsistencies. Additionally, if multiple students have very similar answers, it can indicate the usage of GPT-4 or other AI models.
What makes it challenging for teachers to detect GPT-4 content?
If GPT-4 generated content is produced using advanced prompts or rephrasing tools like QuillBot, or through extensive manual rewriting, it becomes significantly more challenging for teachers to detect GPT-4 content.
What tools can make it harder for teachers to detect GPT-4 content?
Tools like QuillBot can change the sentence structure and wording while preserving the core meaning, making it more challenging for teachers to detect GPT-4 generated content.
How do AI content detectors identify AI-generated content?
AI content detectors analyze language patterns and word choices in the generated text. Metrics like perplexity and burstiness are used to measure the level of randomness or unpredictability in the text. AI-generated content tends to have lower perplexity and randomness scores because the word choices are more predictable.
Can teachers detect GPT-4 content even if it is rephrased?
Even if GPT-4 generated content is rephrased, the essential facts remain the same. This becomes noticeable when multiple students use GPT-4 or any ChatGPT model to generate their content.
What tools can teachers use to detect GPT-4 generated content?
Teachers can use tools like SafeAssign, Turnitin, Copyleaks, and GPTZero to detect GPT-4 generated content and ensure academic integrity.
How are small language models trained on children’s stories?
Small language models are trained on children’s stories to simplify the language and focus on specific tasks. This training allows the models to learn facts, characters, events, and grammar in a simplified context.
What insights can training small language models provide?
Training small language models on children’s stories offers insights into their capabilities and behavior, including word prediction, grammar, and rudimentary logic.
How are small language models evaluated?
Small language models are prompted to generate story endings, and the generated endings are evaluated using grading systems based on criteria like creativity, grammar, and consistency.
What is the Socratic approach to teaching and AI tutoring?
The Socratic approach aims to guide students through the problem-solving process by asking questions that encourage critical thinking. Instead of providing direct answers, the Socratic tutor helps students develop their own understanding and thinking skills.
How do AI models advance detection methods?
As AI models advance, detection methods employed by AI content detectors need to evolve to keep up with changing patterns.
Source Links
- https://trickmenot.ai/can-teachers-detect-gpt4/
- https://www.quantamagazine.org/tiny-language-models-thrive-with-gpt-4-as-a-teacher-20231005/
- https://openai.com/research/gpt-4
I’m Alexios Papaioannou, an experienced affiliate marketer and content creator. With a decade of expertise, I excel in crafting engaging blog posts to boost your brand. My love for running fuels my creativity. Let’s create exceptional content together!